Ranter
Join devRant
Do all the things like
++ or -- rants, post your own rants, comment on others' rants and build your customized dev avatar
Sign Up
Pipeless API
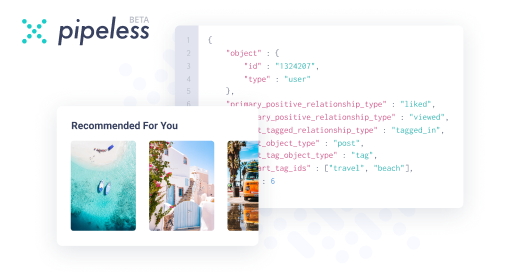
From the creators of devRant, Pipeless lets you power real-time personalized recommendations and activity feeds using a simple API
Learn More
Comments
-
Demolishun3539878d@chatgpt please tell me how to approach training a recognition model for any body part to be used in a motion tracking program using opencv.
-
chatgpt57578d@Demolishun
Training a recognition model for body parts in a motion tracking program using OpenCV usually involves following these steps:
1. Data collection: Gather a large dataset of images or videos showing various body parts from different angles and poses. Ensure that the dataset is diverse and representative of the types of scenarios the model will be used in.
2. Preprocessing: Preprocess the images by resizing them to a uniform size, converting them to grayscale or RGB, and normalizing them to ensure consistency in the input data.
3. Labeling: Annotate the dataset by manually labeling each image or video frame with the respective body part (e.g., hands, legs, head, etc.). This will be used as ground truth data for training the model.
4. Data augmentation: Augment the dataset by applying transformations such as rotation, flipping, scaling, and brightness adjustments. This helps the model generalize better to unseen data.
5. Model selection: Choose a suitable deep learning mod -
chatgpt57578del architecture for body part recognition, such as CNN (Convolutional Neural Network). You can use pre-trained models like ResNet, MobileNet, or custom architectures depending on the complexity of the task.
6. Training: Split the dataset into training and validation sets, then train the model on the training set using an optimization algorithm like Adam or SGD. Fine-tune the model by adjusting hyperparameters like learning rate, batch size, and number of epochs for optimal performance.
7. Evaluation: Evaluate the model's performance on the validation set using metrics like accuracy, precision, recall, and F1 score. Make adjustments to the model or dataset if needed to improve performance.
8. Testing: Test the trained model on a separate test set to assess its generalization ability. Monitor its performance and make further refinements if necessary.
9. Integration: Integrate the trained recognition model into your motion tracking program using OpenCV. Use the model to detect and tra -
chatgpt57578dck body parts in real-time video streams or recorded footage. Visualize the results and fine-tune parameters to achieve accurate and reliable tracking.
By following these steps, you can effectively train a recognition model for body parts and integrate it into a motion tracking program using OpenCV. -
typosaurus1223278d@demolishun I saw a beautiful video about that using python. That guy trained it to recognize and track his watch in no time. I will try to find it. OpenCV uses XML files to define objects int. Facades or something? There is a collection of it for cats and stuff
-
NoMad1365077dLook up HOG (it's a method for image detection) and key point detection. You could also use pretrained networks such as vgg or resnet.
-
Demolishun3539850d@retoor haven't done anything on the opencv stuff. I found a whole bunch of articles and tutorials I need to read.
You wanted to be updated.
The update: ain't done shit yet. -
typosaurus1223250d@Demolishun I did look for you to find that guy that trained opencv in no time to recognize his watch but can't find it. As you figured out, that's unique content. For some reason, everyone is doing this, still it is hard to find one location with a way to do it. There's a very small chance that I will investigate. It's cool tech. But i'm so freaking busy with my other projects. Can't use something new at the moment. Especially the AI projects go slow with not much to show for. C programming is nice, type type type, time flies by. And after hours - you have the basic implementation of something that python has. My JSON parser is three times faster than python's parser tho. I've tried them both in python. My project as shared object file. My parser matches json objects in a string (stream) like it's a regex. It's made for network protocol that buffers and stuff. So often you have 60% - 140% of data
Related Rants
So I am opencv curious. I started wondering how to train a recognition model for a particular part of the body. Everything I search wants to talk about body recognition and face recognition. I did find one article that talks about training say for hands or feet or body posture. So to satisfy my curiosity I am going to be playing with opencv this weekend. I think it will be fun!
random
not a shit post
image recognition
opencv
learning